Technion researchers have developed a microscopic method for creating dynamic XNUMXD images, which is based on deep learning and plans the optical system itself
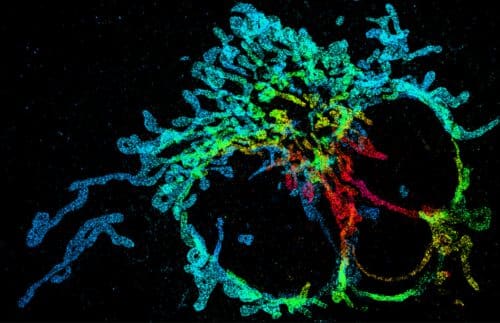
Credit: by courtesy of Nature Methods and authors
Researchers at the Technion present in the journal Nature Methods a leap forward in super-resolution XNUMXD microscopy of cells. The innovative system significantly shortens the time to create the image in XNUMXD, using a neural network and deep learning. The researchers demonstrated the effectiveness of the system experimentally by XNUMXD mapping of mitochondria (the energy producer in the cell) and by tracking telomeres (regions at the ends of chromosomes, responsible among other things for the division of cells in the body) in living cells.
The research was led by Dr. Yoav Shechtman and PhD student Elias Naama from the Faculty of Biomedical Engineering and Dr. Tomer Michaeli from the Faculty of Electrical Engineering at Viterbi.
One of the greatest challenges of biology today is the mapping of dynamic biological processes in living cells at super-resolution, that is, at a resolution 10 times greater than the resolution of a standard optical microscope.
Microscopes, in general, produce 1873D images, but the world is XNUMXD, so XNUMXD information is missing information. XNUMXD images are currently achieved through layered scanning, that is, images of different layers in the sample and their integration by computerized means into a XNUMXD image. The problem is that such a process requires a lot of scanning time, during which the object being studied must be static. In addition, in classical optical microscopy, the level of resolution (the ability to separate) is limited by the "diffraction limit" formulated by the German physicist Ernst Carl Abbe in XNUMX.
This is where DeepSTORM3D comes into the picture - a super-resolution XNUMXD mapping system developed by the researchers. According to Dr. Yoav Shechtman, who led the development of the system, "to obtain depth information from a two-dimensional image, we use wavefront design - an optical method that encodes the depth of each molecule in the form received on the camera. The problem with this method is that when we overlap the fluorescent light points in the sample to get more information, the different shapes overlap each other in the photograph and impair the spatial and temporal resolution."
To face the challenge, the researchers harnessed the hot field of deep learning. They developed an artificial neural network - a system that performs computational tasks at an unprecedented level of performance and speed. Together with Dr. Tomer Michaeli from the Viterbi Faculty of Electrical Engineering, an expert in this field, the researchers developed a neural network that trains on a huge amount of virtual samples and then knows how to analyze the information captured from microscope images of real samples and produce XNUMXD images from it in super- resolution.
According to Dr. Shechtman, "the new technology advances us to the realization of one of the greatest ambitions in biological research - mapping of biological processes in living cells at super-resolution. It is very important to me that people in the life sciences benefit from my equipment, so I maintain close ties with biologists who explain to me what their needs are. It is clear to me that the systems we develop must be friendly enough for users who are not engineers, and we invest effort in this as well in my group."
The neural networks are used by Dr. Shechtman not only in the analysis of the images but also in the improvement of the instruments. "This is perhaps the most exciting future direction that came out of the current development - the neural network provided us with the optimal physical design of the optical system. In other words - the computer not only analyzed the data but told us how to build the microscope. This concept can also be applied in fields not related to microscopy, and we are working on it."
Daniel Friedman from the Google Research Center and researchers and students from the Faculty of Biomedical Engineering, the Lori Lockey Center for Life Sciences and Engineering and the Russell Berry Institute for Nanotechnology at the Technion participated in the study: Racheli Gordon, Boris Fredman, Dr. Lucian Weiss, Dr. Onit Ellof, Tal Naor and Reut Orang '. The study was conducted with the support of the European Research Commission as part of the H2020 program, Google, the National Science Foundation and the Zuckerman Foundation.
One response
The article is in an open version
https://arxiv.org/pdf/1906.09957.pdf